Introduction
Rapid advances in AI, the frenzied deployment of new systems, and the surrounding hype cycle have generated a swell of excitement about AI’s potential to transform society for the better.
But we are not on course to realize those rosy visions. AI’s trajectory is being dictated by a toxic arms race amongst a handful of unaccountable Big Tech companies – surveillance giants who serve as the modern gatekeepers of information, communications, and commerce.
The societal costs of this corporate battle for AI supremacy are already stacking up as companies rush unsafe systems to market – like chatbots prone to confidently spew falsehoods – recklessly integrating them into flagship products and services.
Near-term harms include turbocharging election manipulation and scams, exacerbating bias and discrimination, eroding privacy and autonomy, and many more. And additional systemic threats loom in the medium and longer terms, like steep environmental costs, large-scale workforce disruptions, and further consolidation of power by Big Tech across the digital economy.
Industry leaders have gone even further, warning of the threat of extinction as they publicly echo calls for much-needed regulation – all while privately lobbying against meaningful accountability measures and continuing to release increasingly powerful new AI systems. Given the monumental stakes, blind trust in their benevolence is not an option.
Indeed, a closer examination of the regulatory approaches they’ve embraced – namely ones that forestall action with lengthy processes, hinge on overly complex and hard-to-enforce regimes, and foist the burden of accountability onto those who have already suffered harm – informed the three overarching principles of this Zero Trust AI Governance framework:
- Time is of the essence – start by vigorously enforcing existing laws.
- Bold, easily administrable, bright-line rules are necessary.
- At each phase of the AI system lifecycle, the burden should be on companies to prove their systems are not harmful.
Absent swift federal action to alter the current dynamics – by vigorously enforcing laws on the books, finally passing strong federal privacy legislation and antitrust reforms, and enacting robust new AI accountability measures – the scope and severity of harms will only intensify.
If we want the future of AI to protect civil rights, advance democratic ideals, and improve people’s lives, we must fundamentally change the incentive structure.
Principle 1Time is of the essence – start by vigorously enforcing existing laws.Industry leaders have deployed numerous tactics to cast themselves as thoughtful while delaying accountability. They’ve played up the long-term threat of human extinction, asked Congress to create a new agency, and heaped praise on proposals that would slow-walk action – all while continuing to drive the AI arms race forward at a breakneck speed. But concrete harms from these systems are already being felt, and advancing as rapidly as AI itself. As officials across federal enforcement agencies have underscored, there is no AI exemption from the laws on the books; enforcing them swiftly and vigorously is a critical first step toward mitigating automated harms and deterring the reckless deployment of unsafe systems. |
- Enforce anti-discrimination laws – including the Civil Rights Act, Voting Rights Act, Fair Housing Act, Equal Credit Opportunity Act, Fair Credit Reporting Act, Equal Pay Act, and Americans with Disabilities Act – when AI tools are used to automate unlawful discrimination.
- Enforce consumer protection laws. The FTC has a broad statutory mandate to protect consumers, and they’re already making clear they’ll go after everything from automated scams and false claims about AI tools to deceptive ad practices and privacy abuses. Importantly, they’ve pursued novel remedies that can actually serve as a deterrent, like forcing companies to delete algorithms trained on ill-gotten data.
- Enforce competition laws. Amid the AI arms race, tech giants are already engaging in a wide range of anticompetitive behavior, including, tying and bundling, exclusive dealing, restrictive licensing, and harmful data acquisitions. While Congress should pass new bright-line antitrust laws fit for the digital age, including those outlined in subsequent sections, FTC and DOJ should continue using the totality of their existing authority to confront these abuses, and should utilize their ongoing merger guideline review and the FTC’s commercial surveillance rulemaking to strengthen their hand in combating unfair methods of competition.
- Clarify the limits of Section 230 and support plaintiffs seeking redress for various AI harms. As its authors have made clear, the law shielding digital services from liability for third-party content should not protect generative AI. Defamation cases targeting ChatGPT are already unfolding. More serious cases will surely follow; consider the tragedy in which a chatbot persuaded a man to take his own life, or hypotheticals about providing a deadly recipe. Beyond Section 230, AI companies are being targeted in high-stakes copyright cases, class-action privacy suits, and more. While there are thorny legal questions abound, policymakers should seek opportunities to file amicus briefs and statements of interest in cases that will shape the future of liability for AI-related harms – and the behavior of those who develop and deploy these systems.
Principle 2Bold, easily administrable, bright-line rules are necessary.It should be clear by now that self-regulation will fail to forestall AI harms. The same is true for any regulatory regime that hinges on voluntary compliance or otherwise outsources key aspects of the process to industry. That includes complex frameworks that rely primarily on auditing – especially first-party (internal) or second-party (contracted vendors) auditing – which Big Tech has increasingly embraced. These approaches may be strong on paper, but in practice, they tend to further empower industry leaders, overburden small businesses, and undercut regulators’ ability to properly enforce the letter and spirit of the law. |
- Prohibit unacceptable AI practices. Certain uses of AI are fundamentally incompatible with human rights and should never be permitted, including:
- Emotion recognition or use of biometrics to infer psychological states
- Predictive policing
- Remote biometric identification including use of facial recognition in public spaces
- Social scoring
- Fully automated hiring, firing, and management of workers (including workers classified as independent contractors)
- Prohibit most secondary uses and third-party disclosure of personal data. The failure to pass comprehensive federal privacy legislation in the U.S. has enabled an economy built on surveillance and extraction. Strong data minimization rules that restrict the data firms can collect (and what they can use it for) represent one of the most powerful tools for addressing the toxic dynamics of the AI arms race – from both a privacy perspective and a competition perspective, as Big Tech’s dominance in the space is owed largely to their massive data advantages.
- Prohibit the collection or processing of all sensitive data – as defined in the bipartisan ADPPA – beyond what is strictly necessary to provide or maintain a specific product or service requested by that individual.
- Prohibit biometric data collection or processing in education, workplaces, housing, and hiring.
- Prohibit surveillance advertising.
- Prevent gatekeepers from abusing their power to distort digital markets and perpetuate toxic dynamics in the AI arms race. Cloud infrastructure providers are best placed to reap the advantages as operators of a key bottleneck in building and operating large-scale AI. And the owners of platform ecosystems are positioned to leverage their data advantage and extract rents as these systems are commercialized. Structural interventions are the best way to prevent toxic competition in the market as these companies rush to commercialize AI systems before they’re ready – all to retain first mover advantage.
- Prohibit dominant cloud infrastructure providers from owning or having a beneficial interest in large-scale commercial AI offerings. Large-scale AI models require a tremendous amount of computational power to train and operate, and the $500 billion cloud computing market has been captured by three tech giants: Amazon, Google, and Microsoft. Their dual role as AI leaders and owners of the infrastructure upon which these systems depend is inherently anticompetitive, distorts market incentives, and is perpetuating a toxic arms race, with each company rushing out unsafe AI offerings as they vie for supremacy. America has a long history of enacting structural remedies to separate companies who control critical infrastructure in key distribution networks from business lines that rely on those networks, including in the railroad, banking, and telco industries.
- Prohibit gatekeepers from self-preferencing, boosting business partners, or kneecapping rivals in commercialized AI.
- Prohibit gatekeepers from using non-public data from business users to unfairly compete with them.
Principle 3At each phase of the AI system lifecycle, the burden should be on companies to prove their systems are not harmful.Industry leaders have taken a range of voluntary steps to demonstrate a commitment to key ethical AI principles. But they’ve also slashed AI ethics teams, ignored internal alarms, abandoned transparency as the arms race has escalated, and sought to pass accountability off to downstream users and civil society. Rather than relying on the good will of companies, tasking under-resourced enforcement agencies or afflicted users with proving and preventing harm, or relying on post-market auditing, companies should have to prove their AI offerings are not harmful. |
A Useful Corollary: How High-Risk Products in Other Domains Are Regulated
Given the sweeping societal impacts of advanced AI systems – ones that can unleash widespread harm in the immediate term, according to their makers, and carry existential risk in the longer term – it’s useful to consider how similarly high-risk products are regulated. The development and approval process for bringing new drugs to market is a particularly illuminating corollary.
Pharmaceutical companies spend billions each year on R&D, screening thousands of compounds to identify a few promising candidates for preclinical research, which includes FDA-sanctioned testing on animals and extensive documentation of the drug’s composition and safety. With that research, and detailed protocols for potential clinical trials, they can submit an investigational application to the FDA. If greenlit, they begin the first of three intensive phases of clinical trials, after which they may submit a formal application to bring the drug to market. If the FDA decides to move forward, a review team then evaluates all research to determine if the drug is safe and effective for its intended use. Drugs that are approved – roughly 1-in-10 that entered clinical trials – are then subject to FDA labeling, post-market monitoring, requirements to disclose side effects, and more; any significant changes require supplementary applications for FDA approval.
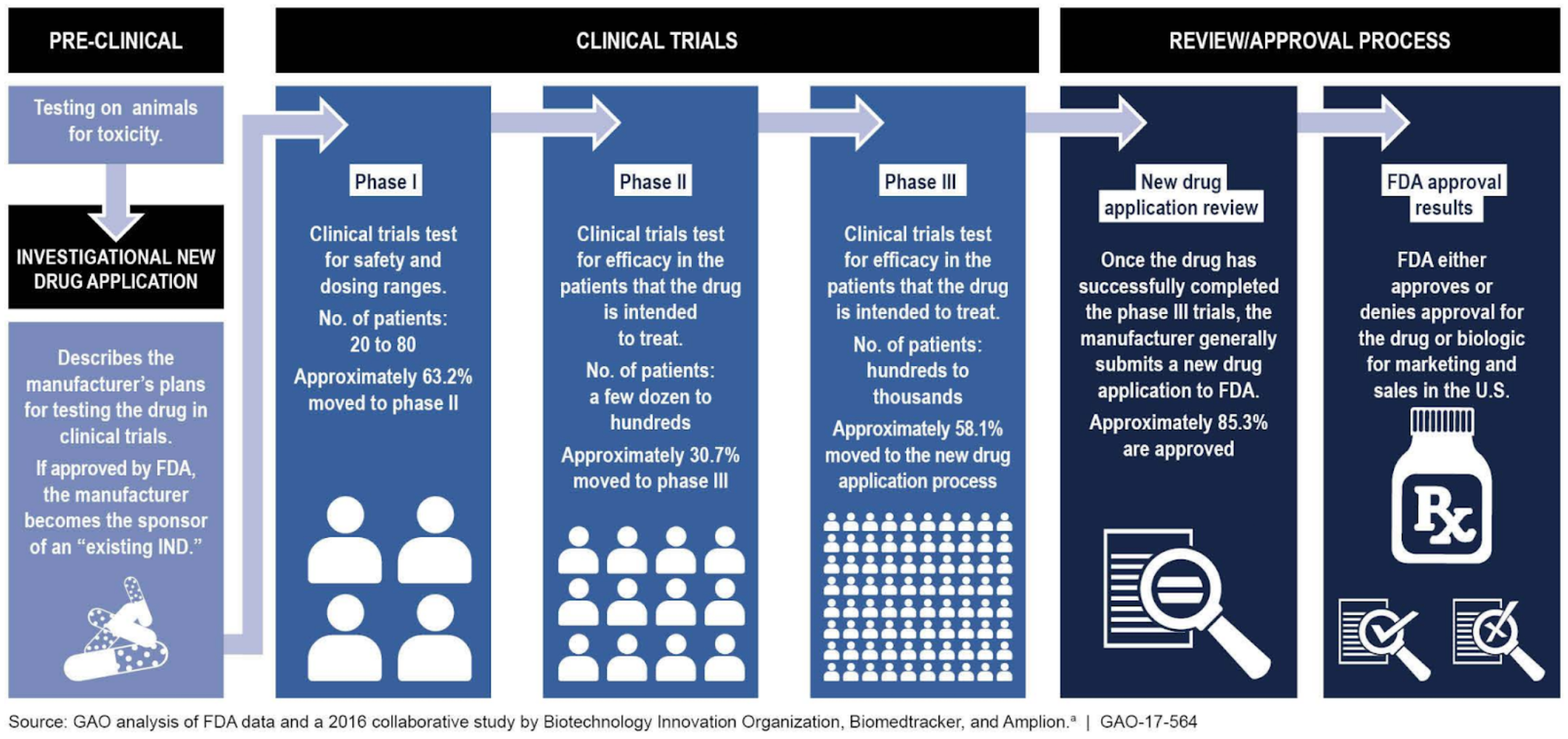
At every stage of the drug development process, companies must adhere to bright-line rules and FDA standards, conduct extensive testing to identify and account for all foreseeable risks, ensure those risks are outweighed by the benefits, and show their work to regulators, knowing that not moving the drug toward market is a likely outcome. They must equip deployers (prescribers) and end users (patients) of their products with clear information about appropriate usage and potential adverse effects. They are liable for harms resulting from failure to fulfill these duties, but not harms driven by prescribers’ negligence or patients’ misuse.
This is not necessarily a call for a new “FDA for AI,” nor is this regime a one-to-one prescription for AI governance, which must be much faster-moving and more elastic, but it’s a helpful guidepost. Large-scale AI models and automated decision systems should similarly be subject to a strong set of pre-deployment requirements.
- Specific standards for evaluation and documentation will necessarily differ depending on the type and maturity of the AI. Consider, for example, an AI tool designed to identify early signs of disease, an automated system used to screen job applicants, and a general-purpose large language model; each offering carries potential to perpetuate serious harms that must be provably accounted for before deployment, but the nature of those risks are divergent and demand substantially different testing.
- There are core AI ethics principles that must be upheld universally, even as the standards for evaluating each system diverge. For example, as recently called for by a group of civil, technology and human rights organizations, the Blueprint for an AI Bill of Rights outlines five categories of core protections that offer a clear roadmap for implementation: safe and effective systems; algorithmic discrimination protections; data privacy; notice and explanation; and human alternatives, considerations, and fallback. These requirements are the floor, not the ceiling. All AI can and should verifiably comply with these principles at a minimum, though compliance will look different for different systems.
- Companies should be required to affirmatively demonstrate compliance with the law throughout all stages of development, appropriate to their role within the supply chain. This should include documenting processes by which companies identify reasonably foreseeable harms, as well as their proposals for how to mitigate them, where that is possible. Given the proliferation of false or exaggerated claims in the AI market, companies must also demonstrate that the systems work as intended (and advertised). Regulators may also require additional testing, inspection, disclosures, or modifications before approval. A public-facing version of all documentation about the system should be published in a federal database upon approval.
Post-deployment requirements should include:
- Ongoing risk monitoring, including via annual civil rights and data privacy impact assessments with independent audits conducted by third-parties with full API and data access, and requirements to maintain an easy complaint mechanism for users and to swiftly report any serious risks that have been identified. Such assessments should be made available to the public in a centralized, accessible repository.
- Proactively notifying users when they are engaging with AI systems, what the intended uses are, and providing them with easily accessible explanations of systems’ main parameters and any opt-out mechanisms or human alternatives available
- Generative AI-specific requirements, including:
-
- Adhering to new provenance, authenticity, and disclosure standards. While far from a panacea for addressing the sweeping harms of generative AI to the information and news ecosystems, establishing effective and interoperable standards for certifying the provenance and authenticity of AI-generated or manipulated media can add critical context that helps protect both consumers and creators of content.
- Bright-line prohibitions on certain unacceptable uses, such as non-consensual dissemination of deepfake sexual images; willfully deceiving a person with the intent of impeding their exercise of the right to vote; or impersonating someone and acting in their assumed character with the intent of obtaining a benefit or injuring or defrauding others.
ConclusionFor too long, we’ve misplaced trust in Big Tech to self-regulate and mistaken technological advances for societal progress, turning a blind eye to the torrent of escalating harms. Zero Trust AI Governance is a necessary course correction as we contend with evolving threats – a framework offering the baseline we need to foster healthy innovation and ensure the next generation of technology serves the greater good. |